When reviewing how advertising performs, there are many metrics to analyze: impressions, clicks, view rates, or increased sales or traffic. But none of those numbers tell the full story of how consumers react to an ad, and how they feel about the message, the product, or the company.
Brands may not know the full story of how their advertisements are perceived, but that is not for lack of data. With each new year in the technological age, we’re creating a compounding amount of data that dwarfs any accumulation in human history. It is estimated that in more data was created in 2017 than in the previous 5000 years of human history.
The trouble with this data is that it is unstructured. The information doesn’t fit a pre-defined model or organization by which we can organize, query, and synthesize it. Most of this unstructured data being created en masse is text-heavy – think social media posts.
Sentiment Analysis is what connects the problem of understanding the qualitative aspects beyond typical engagement measurement, and making sense of the unstructured data that is going unqueried. Sentiment Analysis is a type of natural language processing that utilizes artificial intelligence to determine the attitude or emotion of the author, in regards to a specific topic.
We took the Superbowl as an opportunity to analyze consumer sentiment related to three popular advertisements. A Super Bowl commercial is a sure bet for impressions, but we wanted to know how consumers responded to the ads.
We queried Twitter to find all public tweets related to 3 different Super Bowl advertisements. We took these tweets and fed all of the unstructured text through a natural language processor to review the sentiment. The model analyzes the text and assigns a numerical score that indicates if text is positive or negative. Anything that scores below -0.05 is tagged as negative, and anything about 0.05 is positive. Anything between those numbers is tagged as neutral. The farther away the score gets from neutral indicates the intensity of the emotion. Then the model combines the scores to calculate overall sentiment for a given topic.
Here is what we discovered:
1. Tide’s investment paid off with positive public sentiment.
Tide ads showed up unexpectedly in every quarter, taking over the game by stating that “every ad is a Tide ad” and harkening back on classic ads (think Old Spice and Budweiser). The ad series was funny and lighthearted while being aggressive in establishing its brand presence to make the viewer wonder if all ads are Tide ads. It was also a nice distraction from “eating Tide pods” a recent topic of public derision for the brand.
So how did the sentiment rank?
When we analyzed tweets about the Tide Super Bowl commercial, we saw that when people were talking about this ad also had a positive sentiment for the game, but not as positive as the sentiment for Tide.
Tide Pods also joined the conversation, and the sentiment of Tide Pods during the conversation was overwhelmingly positive.
Tide’s risk-taking and large media buy paid off. The brand is beloved and consumers were happy to speak up about how the commercial brought them joy. Even the thought of eating Tide seems to bring some joy.
2. The public was not afraid to speak up about Dodge Ram in defense of Dr Martin Luther King Jr.
Unfortunately, not all brands who took a risk garnered the same result. Dodge promoted their Ram trucks to the narration of a speech by Dr Martin Luther King Jr, and although they claimed to have worked with the correct parties to get buy-in for the commercial, the public did not appreciate it. The Twittersphere was vocal about their opinions of the ad, and sentiment around Dodge Ram was highly negative.
Notice that beyond the overall sentiment score, there are five different emotions that are also ranked. Disgust and anger were the most strongly expressed emotions around Dodge in response to this commercial.
Interestingly though, there was a clear differentiation between sentiment around the Dodge brand and ad and Dr. Martin Luther King Jr. Sentiment around him remained high, and emotions were mostly joy, anger, and a little sadness.
3. Amazon’s Alexa Loses Her Voice netted positive sentiment for the overall brand and the product.
A much-hyped advertisement for the Super Bowl was a star-studded spot for Amazon about Alexa losing her voice. The ad featured Jeff Bezos himself, and celebrities including Chef Gordon Ramsay, Rebel Wilson, Cadi B and Anthony Hopkins. There was ample media coverage hyping the 90-second spot before it aired.
But how did consumers take it? Overall sentiment for Amazon was slightly positive.
Alexa as her own brand faired higher in consumer sentiment with a higher score. Emotions were primarily joy and sadness.
Understanding sentiment at scale is the tip of the iceberg for AI’s ability to utilize unstructured data to create insights and meaningful connections between brands and consumers. Beyond net sentiment score and emotions, we can gain insights into audience personality, or even analyze tone.
When brands measure performance, putting consumer sentiment at the heart of their performance goals keeps the customer first, and gives more insight into the brand to tell a story beyond the numbers. Brands have the opportunity to utilize data to connect on a more meaningful level with consumers by tapping into feedback. This is an opportunity to go beyond telling a story, to create meaningful connections.
SEEING THROUGH DIFFERENCES
(AT 1/600TH OF A SECOND) Most Idahoans can agree that a learning revolution is critical for Idaho. Of course, we…
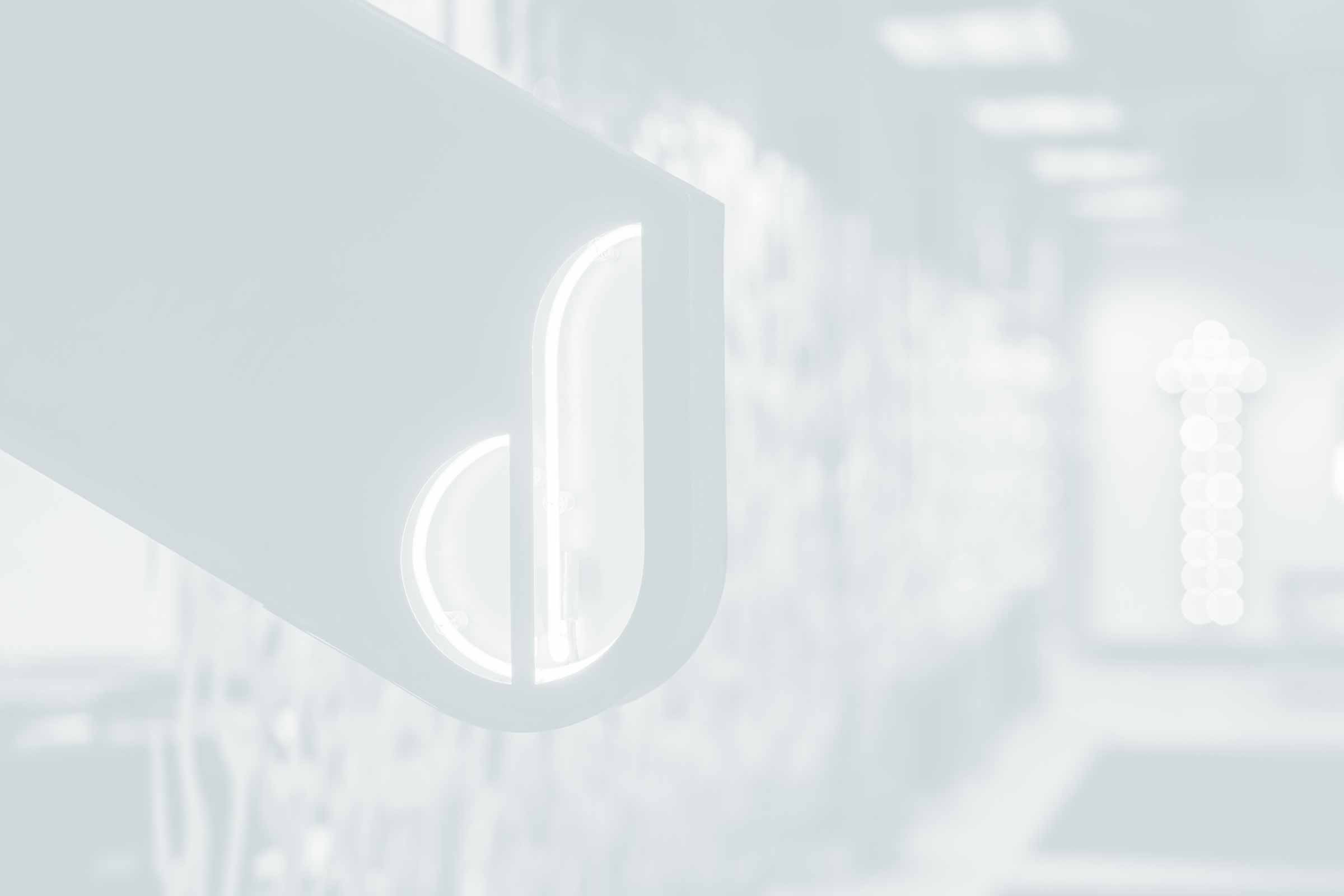
Our Creative Advertising Services
-
Campaign Planning™ & Strategy
Brand & Sales Strategy, Research
-
Art, Story & Experiential
Copywriting, Design, Social, Content
-
UX & Technology
UX, UI, Development, Marketing Automation
-
Findability™
SEO, SEM & Overall User Experience
-
Media Strategy, Planning & Buying
Media Strategy, Planning, Buying, Trafficking & Attribution
-
Data Insights & Analytics
Media Optimization, Trends, Forecasting, Testing
-
Photo & Video Production
Direction, Cinematography, Editing, Post